AI governance refers to the policies, frameworks, and ethical guidelines that dictate how AI systems are developed, deployed, and monitored. Artificial Intelligence (AI) is transforming the world at an unprecedented pace, influencing industries, economies, and even daily human interactions. From automating business operations to enhancing healthcare diagnostics, AI has become an integral part of modern life. However, as AI continues to evolve, so do the challenges and risks associated with its deployment. This is where AI governance comes into play—a structured approach to ensuring AI technologies are used responsibly, ethically, and transparently.
Table of Contents
Definition of AI Governance
AI governance refers to the policies, frameworks, and ethical guidelines that dictate how AI systems are developed, deployed, and monitored. It encompasses regulations, best practices, and accountability measures that ensure AI functions in a way that aligns with societal values and legal standards. Essentially, AI governance seeks to strike a balance between innovation and responsible use, minimizing risks while maximizing AI’s benefits.
Key aspects of AI governance include:
- Ethical considerations – Ensuring AI respects human rights and avoids biases.
- Regulatory compliance – Adhering to laws and industry standards.
- Transparency and explainability – Making AI decisions understandable and accountable.
- Risk management – Identifying and mitigating potential harms of AI applications.
Importance of AI Governance in Today’s AI-Driven World
1. Preventing Bias and Discrimination
AI systems are only as good as the data they are trained on. Without proper governance, AI can inherit and amplify biases, leading to discriminatory outcomes in hiring, lending, policing, and more. AI governance ensures fairness by enforcing guidelines that promote unbiased algorithms and diverse datasets.
2. Enhancing Transparency and Trust
One of the biggest concerns with AI is the “black box” problem—when AI makes decisions without clear explanations. Proper governance demands explainable AI, where users and stakeholders can understand how and why decisions are made, fostering trust in AI-driven systems.
3. Ensuring Compliance with Laws and Regulations
AI operates across industries and borders, making regulatory compliance a necessity. Governance frameworks help organizations comply with data protection laws (such as GDPR or CCPA) and ensure AI applications adhere to legal and ethical standards.
4. Managing AI Risks and Ethical Concerns
AI can be misused for malicious purposes, such as deepfakes, misinformation, or surveillance. Governance frameworks help mitigate these risks by implementing accountability measures and ethical guidelines to prevent misuse.
5. Encouraging Responsible AI Innovation
AI governance is not about restricting progress—it’s about guiding AI development responsibly. With clear regulations and ethical standards, companies can innovate without fear of legal repercussions or public backlash, ensuring AI serves humanity positively.
Why AI Governance is Essential in the Age of Artificial Intelligence
Artificial Intelligence (AI) is revolutionizing industries, streamlining processes, and enhancing decision-making. However, as AI’s influence grows, so do the concerns surrounding its ethical use, potential risks, and accountability. Without proper governance, AI systems can become biased, unregulated, and even harmful to individuals and society. AI governance is the structured approach to ensuring AI operates fairly, ethically, and transparently. But why exactly do we need it? Let’s explore the key reasons AI governance is crucial in today’s world.
Ethical and Moral Considerations
1. Addressing Bias and Discrimination
AI models learn from vast amounts of data, but if that data is flawed, biased, or unrepresentative, the AI can reinforce and amplify discrimination. Examples include biased hiring algorithms that favor certain demographics or facial recognition systems that misidentify minority groups. AI governance establishes ethical guidelines to prevent bias, ensuring fairness and equality in AI-driven decision-making.
2. Protecting Human Rights
AI is being used in areas like law enforcement, hiring, and finance—fields where unfair AI decisions can have life-altering consequences. AI governance helps prevent AI from infringing on human rights by setting ethical boundaries and ensuring AI applications respect individual freedoms and dignity.
3. Preventing Malicious Use of AI
Deepfake technology, AI-generated misinformation, and autonomous weapons pose serious threats to democracy, security, and society. Governance ensures AI is used for constructive purposes while minimizing risks associated with its misuse.
Risk Management and Accountability
1. Avoiding Unintended Consequences
AI systems are complex and sometimes unpredictable. They can make incorrect decisions, act in unintended ways, or be exploited for harmful purposes. AI governance provides a framework to identify, assess, and mitigate risks associated with AI deployment.
2. Establishing Clear Accountability
Who is responsible when AI makes a mistake? If an autonomous vehicle causes an accident, should the blame fall on the manufacturer, the software developer, or the user? Governance establishes accountability structures, ensuring that organizations and individuals take responsibility for AI-driven decisions and their consequences.
3. Regulating AI in Critical Sectors
Industries such as healthcare, finance, and law rely on AI for critical decision-making. Errors in these fields can result in financial ruin, wrongful imprisonment, or even loss of life. Governance ensures that AI systems in high-stakes environments adhere to strict safety, accuracy, and ethical standards.
Trust and Transparency in AI Systems
1. Making AI Decisions Explainable
Many AI systems operate as “black boxes,” making decisions without clear explanations. This lack of transparency creates uncertainty and distrust among users. Governance promotes explainable AI, ensuring that people understand how and why AI makes certain decisions.
2. Building Public Trust in AI
For AI to be widely adopted, people must trust it. When AI is governed effectively—with transparency, fairness, and accountability—it fosters public confidence and willingness to embrace AI-powered solutions in everyday life.
3. Ensuring Regulatory Compliance
Governments and organizations worldwide are establishing AI regulations to prevent unethical AI practices. AI governance helps businesses comply with these evolving regulations, avoiding legal troubles while ensuring responsible AI usage.
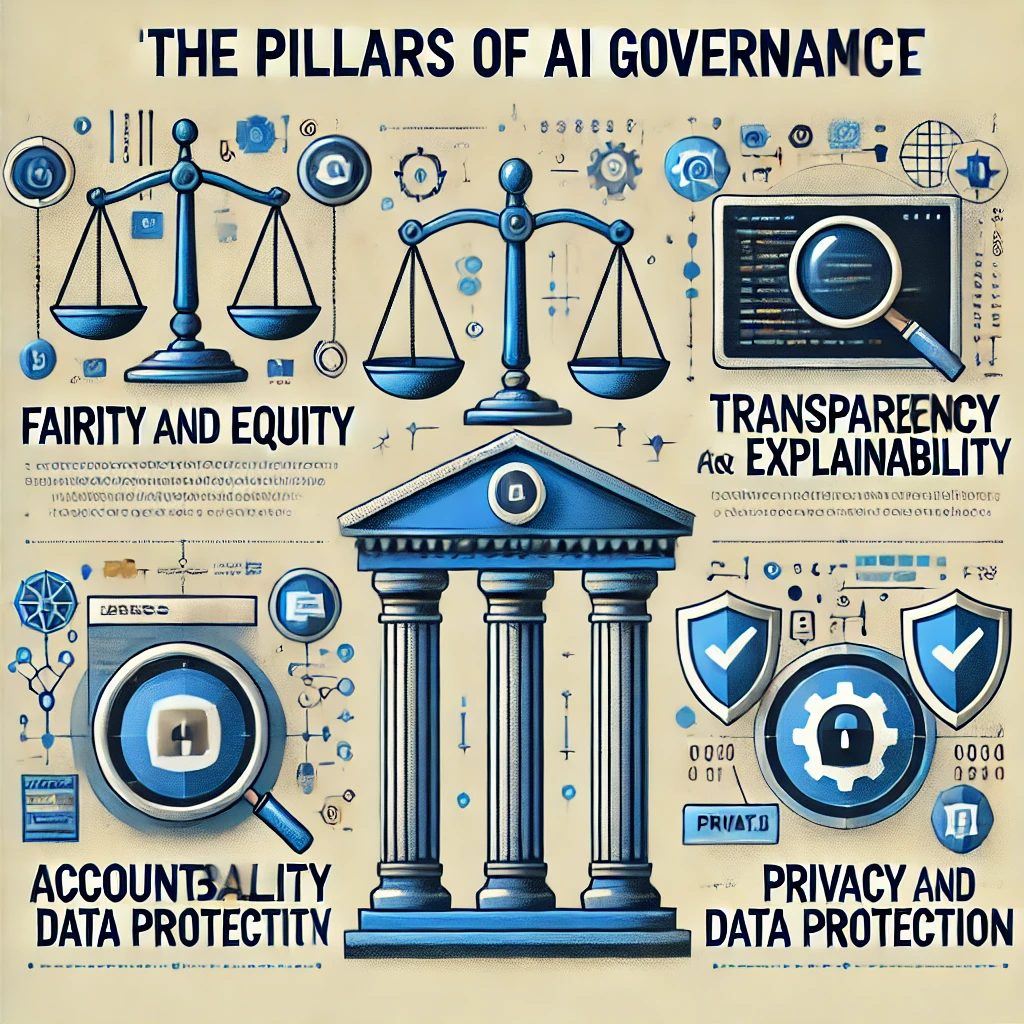
The Pillars of AI Governance: Building a Responsible AI Future
As Artificial Intelligence (AI) continues to reshape industries and societies, ensuring its responsible and ethical use has never been more critical. AI governance serves as the foundation for guiding AI’s development and deployment in a way that aligns with human values, legal standards, and societal expectations. But what exactly makes AI governance effective?
At its core, AI governance rests on four key pillars: Fairness and Equity, Transparency and Explainability, Accountability and Oversight, and Privacy and Data Protection. These principles ensure that AI systems operate ethically, responsibly, and in the best interests of all. Let’s explore each of these pillars and why they are essential for the future of AI.
1. Fairness and Equity
AI systems are only as unbiased as the data they are trained on. Without careful oversight, AI can perpetuate or even amplify biases present in historical data, leading to unfair outcomes.
Why Fairness and Equity Matter
- AI is used in hiring, lending, healthcare, and law enforcement—areas where biased decisions can have severe consequences.
- Certain demographics may be disproportionately disadvantaged if AI systems reinforce existing inequalities.
- AI should provide equal opportunities for all, regardless of race, gender, or socioeconomic background.
How AI Governance Ensures Fairness
- Bias Audits: Regular evaluations of AI systems to detect and mitigate biases.
- Diverse Training Data: Ensuring AI models are trained on inclusive, representative datasets.
- Fairness Standards: Developing and enforcing guidelines to prevent discrimination in AI-driven decision-making.
By prioritizing fairness, AI governance helps build systems that treat all individuals equitably, reducing disparities rather than reinforcing them.
2. Transparency and Explainability
Many AI systems function as “black boxes,” making decisions without clear explanations. This lack of transparency creates mistrust and raises ethical concerns, particularly in high-stakes applications like medical diagnoses and criminal justice.
Why Transparency and Explainability Matter
- Users and stakeholders need to understand how and why AI systems make decisions to trust their reliability.
- Lack of transparency can lead to hidden biases, unfair practices, and ethical dilemmas.
- Regulatory bodies and users should be able to audit AI systems to ensure compliance with ethical standards.
How AI Governance Promotes Transparency
- Explainable AI (XAI): Encouraging the development of AI models that can articulate their decision-making process in a human-understandable way.
- Open AI Policies: Organizations should disclose how their AI models work, the data they use, and the logic behind key decisions.
- User Awareness: Informing users when they are interacting with AI and providing them with clear explanations of AI-driven outcomes.
Transparency ensures that AI systems are not just powerful but also trustworthy and accountable to those they serve.
3. Accountability and Oversight
When AI makes a mistake—whether it’s a wrongful loan rejection, an incorrect medical diagnosis, or biased law enforcement profiling—who is responsible? Without proper accountability, organizations may evade responsibility, leading to harmful and unchecked AI use.
Why Accountability and Oversight Matter
- AI decisions can have real-life consequences that impact individuals and communities.
- Organizations and developers must be held responsible for the outcomes of their AI systems.
- Clear governance ensures AI is developed and used in a way that aligns with legal and ethical frameworks.
How AI Governance Ensures Accountability
- Human-in-the-Loop (HITL) Systems: Keeping human oversight in AI decision-making processes to prevent catastrophic errors.
- Clear Legal Frameworks: Establishing regulations that outline liability for AI-driven decisions.
- Independent Audits: Third-party reviews of AI systems to ensure compliance with ethical and legal standards.
By enforcing accountability, AI governance ensures that AI systems operate within a structured framework where developers, companies, and regulators take responsibility for AI’s impact.
4. Privacy and Data Protection
AI thrives on data, but how that data is collected, stored, and used raises major privacy concerns. Without proper governance, AI can infringe on user privacy, leading to data breaches, surveillance overreach, and misuse of personal information.
Why Privacy and Data Protection Matter
- AI systems often process vast amounts of sensitive personal data, making them potential targets for cyber threats.
- Users should have control over their data and the ability to opt out of intrusive AI-driven tracking.
- Data misuse can lead to identity theft, discrimination, and erosion of personal freedoms.
How AI Governance Protects Privacy
- Data Minimization: AI should only collect and store the data it truly needs.
- Regulatory Compliance: Organizations must follow data protection laws such as GDPR (Europe) and CCPA (California).
- Encryption and Security Measures: Protecting data from unauthorized access and cyber threats.
Ensuring privacy and data protection safeguards individuals from AI-driven exploitation, fostering trust and security in AI applications.
Principles and Standards of Responsible AI Governance
Artificial Intelligence (AI) is rapidly becoming a fundamental part of our lives, influencing everything from healthcare and finance to education and law enforcement. While AI presents incredible opportunities, it also comes with significant ethical and regulatory challenges. Ensuring AI is used responsibly, ethically, and transparently is essential to building public trust and minimizing harm.
This is where AI governance comes in—providing the necessary frameworks, principles, and standards that guide the responsible development and deployment of AI. But what does responsible AI governance look like in practice? In this post, we’ll explore key frameworks, ethical guidelines, and core principles that form the foundation of AI governance.
Frameworks and Guidelines for Ethical AI
To ensure AI aligns with human values and societal norms, various organizations, governments, and research institutions have developed ethical AI frameworks. These frameworks serve as blueprints for organizations to follow, ensuring AI is developed and deployed in a way that is fair, accountable, and transparent.
1. International AI Governance Initiatives
Several global organizations have established AI governance frameworks to promote ethical AI use:
- OECD AI Principles (Organization for Economic Co-operation and Development)
- Emphasizes inclusive growth, human-centered values, transparency, robustness, and accountability.
- Serves as a guideline for policymakers in shaping responsible AI strategies.
- EU AI Act (European Union)
- Introduces a risk-based classification of AI systems, with stricter regulations for high-risk applications.
- Focuses on safety, human oversight, and legal compliance.
- NIST AI Risk Management Framework (National Institute of Standards and Technology, USA)
- Encourages organizations to implement risk-based approaches to AI development.
- Highlights explainability, fairness, and security as core components.
2. Corporate AI Ethics Guidelines
Tech giants and research institutions have also developed internal AI governance frameworks:
- Google’s AI Principles – Focuses on social benefits, avoiding bias, and ensuring safety.
- Microsoft’s Responsible AI Standards – Highlights transparency, privacy, and accountability.
- IBM’s AI Ethics Board – Implements AI fairness and responsible data use policies.
These frameworks ensure that AI development is aligned with ethical principles while allowing organizations to innovate responsibly.
Key Principles of Responsible AI Governance
While AI governance frameworks may vary, three core principles remain universal: Fairness, Accountability, and Transparency. These principles form the ethical foundation for AI regulation and ensure AI systems operate in a way that benefits society.
1. Fairness: Preventing Bias and Discrimination
AI must be fair and inclusive, ensuring that all individuals—regardless of race, gender, or socioeconomic background—are treated equitably.
Challenges of Fairness in AI:
- AI models trained on biased data can reinforce discrimination in hiring, lending, and law enforcement.
- Some AI systems disproportionately favor certain groups over others, leading to unethical outcomes.
How Governance Ensures Fairness:
- Bias Detection Tools – Implementing fairness audits to detect and mitigate bias in AI models.
- Diverse Training Data – Ensuring AI systems are trained on inclusive datasets to prevent discrimination.
- Fairness Standards – Creating guidelines that require AI systems to make equitable and non-discriminatorydecisions.
Fairness in AI governance ensures that AI serves everyone equally, rather than benefiting only certain groups.
2. Accountability: Who Is Responsible for AI Decisions?
With AI increasingly making critical decisions, who should be held accountable when AI makes a mistake? Without clear accountability, AI risks causing harm without anyone taking responsibility.
Challenges of AI Accountability:
- Autonomous AI decisions can impact lives, but responsibility is often unclear.
- Companies may shift blame onto AI models, avoiding legal or ethical consequences.
- Legal systems worldwide lack clear frameworks for determining AI accountability.
How Governance Ensures Accountability:
- Human-in-the-Loop (HITL) Systems – Ensuring human oversight in critical AI decision-making.
- Legal Liability Frameworks – Defining legal accountability for AI-driven mistakes.
- Independent AI Audits – Requiring organizations to regularly assess AI performance and impacts.
By enforcing accountability, AI governance ensures that companies and developers take responsibility for AI’s consequences, preventing harm and misuse.
3. Transparency: Making AI Understandable
AI models, especially deep learning systems, often operate as black boxes, making decisions that even their developers can’t fully explain. Transparency ensures that AI decisions are clear, explainable, and open to scrutiny.
Challenges of AI Transparency:
- Many AI systems make decisions in ways that are not understandable to users.
- Lack of transparency creates mistrust in AI-driven systems.
- Users have limited ability to question or challenge AI decisions.
How Governance Ensures Transparency:
- Explainable AI (XAI) – Designing AI models that clearly justify their decisions.
- Open AI Policies – Requiring organizations to disclose how AI models work, what data they use, and how decisions are made.
- User Awareness – Informing users when they are interacting with AI and allowing them to request explanations.
By making AI systems more transparent, governance frameworks ensure AI can be trusted, understood, and held accountable.
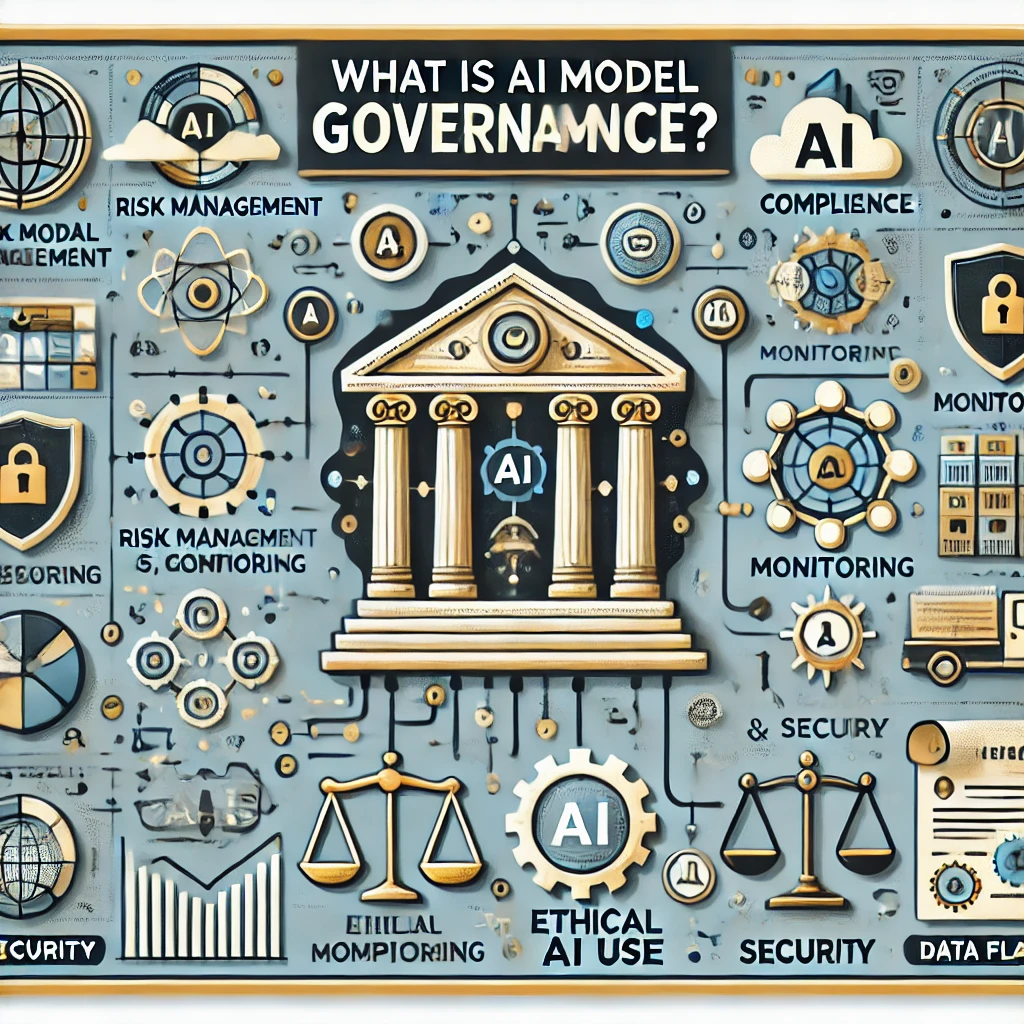
What is AI Model Governance?
Artificial Intelligence (AI) is revolutionizing industries by making faster, data-driven decisions in areas like healthcare, finance, and law enforcement. However, with great power comes great responsibility. AI models can be biased, opaque, and unpredictable, leading to ethical dilemmas, regulatory challenges, and unintended consequences.
This is where AI model governance comes in. AI model governance is the structured approach to overseeing, managing, and regulating AI models to ensure they function ethically, transparently, and in compliance with legal standards. It ensures AI systems remain accountable, explainable, and free from harmful biases.
Let’s dive into the key aspects of AI model governance and why it’s critical for responsible AI development.
Ensuring AI Models Align with Ethical and Regulatory Requirements
AI models don’t operate in isolation—they interact with people, businesses, and governments, often making critical decisions. Without proper governance, AI can lead to biased hiring practices, unfair loan approvals, and unethical surveillance.
Why Ethical and Regulatory Compliance Matters
- AI bias can lead to discrimination, particularly in sensitive applications like hiring, credit scoring, and criminal justice.
- AI must comply with laws and regulations, such as GDPR (General Data Protection Regulation) and the EU AI Act, to ensure ethical use.
- Transparency and fairness are essential to building public trust in AI-driven systems.
How AI Model Governance Ensures Compliance
- Establishing Ethical Standards
- Organizations must define ethical AI principles, such as fairness, transparency, and human oversight.
- AI models should align with human rights laws and anti-discrimination policies.
- Regulatory Alignment
- AI systems must follow global and industry-specific regulations, such as:
- GDPR (Europe): Protecting personal data and privacy in AI applications.
- EU AI Act: Categorizing AI systems based on risk and imposing strict requirements for high-risk applications.
- NIST AI Risk Management Framework (USA): Promoting risk-based AI governance.
- AI systems must follow global and industry-specific regulations, such as:
- Continuous Monitoring and Audits
- AI models must be regularly reviewed and tested to ensure compliance with ethical guidelines.
- Organizations should implement independent AI audits to assess potential risks and biases.
By ensuring AI models align with ethical and legal standards, AI governance prevents harm, builds trust, and ensures responsible innovation.
Managing Risk and Ensuring Explainability
One of the biggest challenges in AI governance is managing risks and ensuring AI decisions are explainable. AI models, particularly deep learning systems, can function as black boxes, making decisions that even developers struggle to interpret.
Why Risk Management and Explainability Matter
- AI models can make incorrect or biased decisions, leading to financial losses, reputational damage, and legal consequences.
- A lack of explainability makes it difficult to understand AI decisions, leading to distrust and ethical concerns.
- High-risk AI applications (e.g., autonomous vehicles, medical diagnostics) require clear accountability and human oversight.
How AI Model Governance Addresses These Challenges
- Risk Management Frameworks
- Organizations should use frameworks like NIST’s AI Risk Management Model to identify, assess, and mitigate AI-related risks.
- Implement risk categorization (low, medium, high risk) to determine how much oversight an AI model needs.
- Explainable AI (XAI) Techniques
- AI models must be designed with explainability in mind, ensuring humans can understand how decisions are made.
- Techniques like SHAP (Shapley Additive Explanations) and LIME (Local Interpretable Model-Agnostic Explanations) help make AI predictions more interpretable.
- Human-in-the-Loop (HITL) Oversight
- AI should not make autonomous high-stakes decisions without human review.
- Governance policies should require human oversight in critical areas like healthcare, finance, and law enforcement.
- AI Performance Monitoring
- AI models should be continuously tested and updated to detect issues such as drift, bias, or unexpected behavior.
- Organizations should establish AI ethics boards or governance teams to oversee AI performance.
By managing risks and ensuring explainability, AI model governance reduces unintended harm, increases transparency, and improves accountability in AI decision-making.
Examples of AI Governance in Action
AI governance is not just a theoretical concept—it is being actively implemented by organizations, governments, and industries worldwide. Companies and policymakers are developing governance frameworks, enforcing ethical AI standards, and ensuring compliance with regulations to prevent AI-related risks and build public trust.
Case Studies of Organizations Implementing AI Governance Frameworks
1. Microsoft’s Responsible AI Framework
Microsoft has been a leader in AI governance, developing a comprehensive framework to ensure its AI systems are fair, transparent, and accountable.
Key AI Governance Practices at Microsoft:
- Responsible AI Committee: Oversees AI ethics and compliance across the company.
- Fairness Audits: Microsoft tests AI models for bias and discrimination, particularly in areas like facial recognition.
- Transparency Standards: AI models must provide clear explanations for their decisions, especially in high-risk applications.
Impact:
- Microsoft discontinued its emotion recognition AI due to concerns about accuracy and ethical implications.
- The company developed Azure AI Responsible AI Tools, enabling developers to build fair and interpretable AI models.
2. Google’s AI Principles and Governance Strategy
Google established a set of AI Principles to guide ethical AI development, focusing on fairness, privacy, and user safety.
Key AI Governance Practices at Google:
- Internal AI Ethics Review Board: Evaluates AI projects for ethical risks.
- Explainability and Fairness Tools: Open-source tools like TCAV (Testing with Concept Activation Vectors)help ensure transparency in AI decisions.
- AI Governance in Hiring: Google improved its hiring AI by ensuring diverse and unbiased training data.
Impact:
- Google shut down Project Maven, a military AI project, after employee protests about ethical concerns.
- The company developed Explainable AI (XAI) tools to help users understand how Google’s AI models make decisions.
3. IBM’s AI Ethics Board and Governance Framework
IBM has taken a proactive approach to AI governance by embedding ethics into AI development through its AI Ethics Board.
Key AI Governance Practices at IBM:
- Watson OpenScale: A tool that monitors AI models for bias and fairness in real-time.
- Transparency in AI Development: IBM publishes research on AI ethics and bias mitigation.
- Privacy-First AI Approach: AI systems follow strict data protection guidelines, ensuring compliance with laws like GDPR.
Impact:
- IBM stopped selling facial recognition technology due to concerns about racial bias and misuse by law enforcement.
- IBM’s AI governance tools are used by enterprises and government agencies to ensure compliance and ethical AI use.
4. EU AI Act: A Government-Led AI Governance Initiative
The European Union AI Act is one of the most comprehensive AI governance frameworks, classifying AI systems by risk and setting strict compliance rules.
Key Governance Features of the EU AI Act:
- Risk-Based Classification:
- Unacceptable Risk AI (e.g., social scoring systems) is banned.
- High-Risk AI (e.g., in healthcare, finance, and law enforcement) requires strict oversight.
- Transparency Requirements: AI systems must be explainable, particularly in high-risk applications.
- Accountability Rules: Organizations deploying AI must prove compliance with ethical guidelines.
Impact:
- The EU AI Act has set a global precedent for AI governance, influencing regulations in the U.S., Canada, and Asia.
- Companies operating in Europe must now ensure AI transparency, fairness, and risk management.
Examples of Successful AI Governance Initiatives
1. AI Governance in Healthcare: FDA’s AI Regulations
The U.S. Food and Drug Administration (FDA) has developed AI governance frameworks for medical AI applications, ensuring patient safety and ethical use.
Key AI Governance Actions:
- Real-World Performance Monitoring: AI medical tools must be continuously monitored for accuracy and safety.
- Transparency Rules: AI models used for diagnosis must explain their reasoning to doctors and patients.
- Risk-Based Oversight: AI models in high-risk areas, like cancer detection, require rigorous validation before approval.
Impact:
- AI-powered diagnostic tools now require human-in-the-loop oversight, ensuring doctors make final decisions.
- The FDA’s AI governance framework has improved patient trust in AI-assisted healthcare.
2. AI Governance in Finance: JPMorgan’s AI Fairness Initiative
JPMorgan Chase, one of the largest financial institutions, has implemented an AI governance framework to ensure fairness and accountability in its AI-driven financial services.
Key AI Governance Actions:
- Bias Audits for AI Credit Scoring: Ensures AI-driven loan approvals do not discriminate based on race or gender.
- Explainability Standards: Customers can request explanations for AI-driven financial decisions.
- AI Risk Management: AI models undergo regular compliance checks to align with financial regulations.
Impact:
- JPMorgan’s AI governance framework prevented biased lending practices, improving trust in AI-powered financial services.
- The bank’s AI transparency policies set a benchmark for ethical AI in banking.
3. AI Governance in Hiring: LinkedIn’s Fairness and Bias Mitigation
LinkedIn, the world’s largest professional networking platform, has taken significant steps to reduce AI bias in hiring and recruitment.
Key AI Governance Actions:
- Bias Detection Algorithms: LinkedIn’s AI monitors job recommendation algorithms for unfair patterns.
- Diversity-Inclusive AI Training: AI models are trained on diverse candidate data to prevent discrimination.
- Transparency Reports: LinkedIn publishes reports on how AI-driven hiring tools operate and their fairness levels.
Impact:
- LinkedIn improved fairness in job recommendations, helping reduce gender and racial bias in AI-powered hiring.
- The company’s AI governance model has become an industry standard for ethical AI use in recruitment.
How Organizations Should Approach AI Governance
AI is transforming industries, but its rapid adoption comes with ethical, legal, and operational challenges. Organizations that develop or deploy AI must establish strong governance frameworks to ensure fairness, transparency, and accountability. Without proper oversight, AI can lead to biased decisions, privacy violations, and legal risks, damaging public trust and corporate reputations.
1. Building Internal AI Governance Structures
AI governance must be embedded into an organization’s culture and operations to ensure that AI systems align with ethical principles and regulatory requirements. A strong internal governance structure provides clear accountability and oversight.
Key Steps to Establish Internal AI Governance:
A. Define AI Governance Policies
- Develop AI ethics guidelines aligned with legal and industry standards.
- Set clear policies on bias mitigation, transparency, and data privacy.
- Ensure AI governance is integrated into existing risk management frameworks.
B. Establish an AI Governance Board or Committee
- Create a cross-functional team with representatives from legal, compliance, data science, and ethics departments.
- The board should review AI projects, assess risks, and enforce compliance.
- Regularly update AI governance policies based on technological advancements and regulatory changes.
C. Implement AI Risk Assessment and Auditing
- Require AI models to undergo regular fairness and bias audits.
- Establish risk classification frameworks (e.g., low-risk, high-risk AI applications).
- Use third-party auditors to evaluate AI transparency and accountability.
D. Ensure AI Model Explainability and Transparency
- Require explainable AI (XAI) methods to make AI decisions understandable.
- Provide clear documentation on how AI models work and what data they use.
- Develop user-friendly AI transparency reports for internal teams and external regulators.
By building a robust internal governance structure, organizations can ensure AI is developed, deployed, and monitored responsibly.
2. Engaging Stakeholders and Fostering Collaboration
AI governance isn’t just an internal effort—it requires collaboration between stakeholders, including employees, customers, regulators, and industry partners. Effective engagement builds trust, accountability, and shared responsibility for ethical AI use.
Key Strategies for Stakeholder Engagement:
A. Internal Engagement: Educating Employees on AI Ethics
- Provide AI ethics training to employees across departments.
- Encourage AI developers and data scientists to follow ethical design principles.
- Establish AI ethics reporting channels for employees to raise concerns.
B. External Engagement: Collaborating with Regulators and Industry Groups
- Work with regulatory bodies to ensure compliance with evolving AI laws.
- Participate in AI ethics research initiatives to contribute to best practices.
- Join industry consortiums, such as the Partnership on AI and the OECD AI Policy Observatory, to share AI governance insights.
C. Public Engagement: Building Trust with Customers and Communities
- Be transparent about how AI is used in products and services.
- Allow users to opt-out of AI-driven decisions when possible.
- Engage with civil society groups to address AI’s impact on human rights and equity.
By involving all stakeholders in AI governance, organizations can create more ethical, inclusive, and responsible AI systems.
3. Evaluating the Impact of AI on Human and Societal Well-Being
AI governance must go beyond compliance—it should assess AI’s broader impact on people, communities, and society. Organizations should continuously monitor AI’s real-world effects and adapt governance policies accordingly.
Key Methods for Evaluating AI’s Societal Impact:
A. Conduct AI Impact Assessments
- Use AI ethics impact assessments (AIEIA) to evaluate how AI affects individuals and communities.
- Assess potential biases, economic disruptions, and social inequalities caused by AI systems.
- Implement safeguards for vulnerable populations affected by AI-driven decisions.
B. Ensure AI Aligns with Human Rights and Ethical Principles
- Follow global ethical AI frameworks, such as UNESCO’s AI Ethics Guidelines.
- Avoid deploying AI in ways that violate privacy, freedom, or equity.
- Support AI for good initiatives, such as AI-driven solutions for climate change, education, and healthcare.
C. Continuously Monitor AI’s Long-Term Effects
- Establish feedback loops to track AI’s real-world performance over time.
- Regularly update AI governance policies based on new research and emerging risks.
- Adapt AI models to changing societal values, cultural norms, and legal standards.
By prioritizing human and societal well-being, organizations can ensure AI is a force for positive change rather than harm.
The Role of Data Governance in AI Governance
AI governance and data governance are deeply interconnected. AI models are only as good as the data they are trained on, making data quality, security, and compliance essential for responsible AI. Without proper data governance, AI systems can become biased, inaccurate, insecure, and legally non-compliant, leading to unethical decisions, privacy violations, and regulatory penalties.
1. Data Quality, Security, and Lineage: The Foundations of AI Governance
Data governance ensures that data used in AI models is accurate, secure, and ethically sourced. These three pillars—quality, security, and lineage—are essential to AI governance.
A. Data Quality: Ensuring AI Models are Accurate and Fair
AI systems rely on large datasets to make predictions, automate decisions, and learn patterns. Poor data quality leads to biased, misleading, or even harmful AI outcomes.
Key Practices for High-Quality Data:
- Consistency & Accuracy: Ensure data is complete, clean, and up-to-date.
- Bias & Fairness Checks: Audit datasets for imbalanced or discriminatory patterns.
- Standardization: Implement uniform data formats and definitions across all AI projects.
Example:
If an AI-powered hiring tool is trained on biased historical hiring data, it may favor certain demographics over others. Proper data governance ensures fair representation of candidates to prevent discrimination.
B. Data Security: Protecting AI Systems from Cyber Threats
AI models process sensitive personal and business data, making data security a critical part of AI governance. Unauthorized access, data leaks, or cyberattacks can compromise AI models and expose organizations to legal risks.
Key Data Security Measures:
- Encryption: Protect AI training data using strong encryption protocols.
- Access Controls: Restrict AI dataset access to authorized personnel only.
- Data Masking & Anonymization: Remove personally identifiable information (PII) from AI datasets.
Example:
A healthcare AI system analyzing patient data must comply with HIPAA (Health Insurance Portability and Accountability Act) regulations to prevent unauthorized access to medical records.
C. Data Lineage: Tracking Where Data Comes From and How It’s Used
Data lineage refers to tracking the origin, transformations, and movement of data throughout its lifecycle. This ensures AI models use reliable, traceable, and compliant data sources.
Key Data Lineage Practices:
- Metadata Management: Maintain detailed records of where data originates and how it has been modified.
- Automated Tracking Tools: Use AI-driven tools to trace data flows and transformations.
- Auditability: Ensure data usage is transparent and accountable for compliance audits.
Example:
A financial institution using AI for credit scoring must track the sources of customer data to ensure compliance with GDPR and other financial regulations.
By enforcing high data quality, security, and lineage, organizations strengthen AI governance and mitigate risks.
2. Aligning Data Practices with AI Governance Objectives
To ensure AI governance is effective, data governance must be fully aligned with ethical AI principles, regulatory requirements, and business goals.
A. Aligning Data Governance with Ethical AI Principles
Data governance should uphold the core ethical principles of AI governance, such as:
- Fairness: Ensure AI datasets represent diverse populations to avoid bias.
- Transparency: Provide clear documentation on data sources and AI decision-making processes.
- Accountability: Assign ownership and responsibility for data integrity and compliance.
Example:
A bank using AI for loan approvals must ensure its customer data is fair and unbiased so the AI does not unintentionally discriminate against minority groups.
B. Regulatory Compliance: Ensuring AI Models Follow Data Protection Laws
AI must comply with global data regulations, such as:
- GDPR (Europe): Ensures AI models handle personal data lawfully, fairly, and transparently.
- CCPA (California): Requires companies to disclose how AI models use consumer data.
- AI Act (EU): Classifies AI systems by risk level, enforcing strict data protection requirements for high-risk AI.
Key Compliance Strategies:
- Implement data privacy impact assessments (DPIAs) for AI projects.
- Ensure data retention policies comply with legal mandates.
- Allow users to opt-out of AI-driven decisions affecting them.
Example:
An AI-powered customer service chatbot that collects user data must comply with GDPR’s “right to be forgotten”, allowing users to request data deletion.
C. Data Governance for AI Risk Management
AI systems pose risks such as bias, privacy breaches, and incorrect predictions. Strong data governance helps identify, mitigate, and monitor these risks.
Risk Management Strategies:
- Bias Detection Tools: Use AI governance tools like IBM Watson OpenScale to detect bias in datasets.
- AI Model Audits: Conduct regular third-party audits to ensure AI models make fair and accurate decisions.
- Incident Response Plans: Establish procedures to correct AI model errors or biases when detected.
Example:
If an AI-driven fraud detection system mistakenly flags legitimate transactions as fraud due to biased training data, data governance ensures rapid detection and correction of such errors.
By aligning data governance with AI governance objectives, organizations create ethical, reliable, and compliant AI systems.
Navigating AI Governance Regulations
As AI technologies advance, governments worldwide are enacting regulations to ensure responsible development and deployment. These regulations aim to prevent bias, protect privacy, and ensure transparency while allowing innovation to thrive. However, the global AI regulatory landscape is fragmented, with different countries adopting varying approaches.
1. Overview of Global Regulatory Frameworks
A. The EU AI Act: The World’s Most Comprehensive AI Regulation
The EU AI Act, proposed in 2021 and expected to take full effect by 2026, is the most detailed AI regulation to date. It classifies AI systems based on risk levels and imposes strict compliance measures.
Key Features of the EU AI Act:
- Risk-Based Classification:
- Unacceptable Risk AI: Banned (e.g., social scoring, subliminal manipulation).
- High-Risk AI: Strict oversight (e.g., AI in hiring, law enforcement, healthcare).
- Limited-Risk AI: Transparency requirements (e.g., chatbots must disclose they are AI).
- Minimal-Risk AI: No restrictions (e.g., AI for gaming or spam filters).
- Transparency & Explainability: High-risk AI systems must provide clear explanations of their decisions.
- Accountability & Human Oversight: High-risk AI applications require human monitoring and intervention.
- Fines for Non-Compliance: Up to €30 million or 6% of global revenue for violations.
Impact on Organizations:
- Companies operating in the EU or serving EU customers must comply.
- Businesses must conduct AI risk assessments before deployment.
- AI models must have documentation proving fairness, accuracy, and security.
B. OECD AI Principles: A Global Ethical AI Framework
The OECD AI Principles, adopted by 42 countries, provide a global baseline for ethical AI governance. While not legally binding, they influence regulations worldwide, including the EU AI Act and U.S. policies.
Key OECD AI Principles:
- Human-Centered AI: AI should respect human rights and democracy.
- Fairness & Accountability: AI systems must prevent bias and discrimination.
- Transparency & Explainability: AI decisions should be understandable.
- Robustness & Security: AI must be resilient against risks.
Impact on Organizations:
- Companies can align internal AI policies with OECD principles for ethical AI adoption.
- OECD standards often influence national AI regulations, making compliance beneficial for global businesses.
C. The U.S. and Canada’s AI Regulatory Approaches
U.S. AI Governance: A Decentralized Approach
The U.S. has no single federal AI law but enforces AI governance through:
- The White House AI Bill of Rights (2022): A non-binding guideline promoting AI fairness, privacy, and transparency.
- Sector-Specific AI Rules:
- Healthcare: FDA regulates AI in medical devices.
- Finance: AI in banking must comply with Fair Lending Laws.
- Employment: AI hiring tools must avoid discrimination under EEOC guidelines.
- State-Level Regulations:
- California Consumer Privacy Act (CCPA): AI-driven data collection must be transparent.
- New York Bias Audit Law: AI hiring tools must pass fairness audits.
Impact on Organizations:
- Companies using AI in healthcare, finance, and employment must ensure compliance.
- AI explainability and bias audits are increasingly required in the U.S.
Canada’s AI Regulation: The Artificial Intelligence and Data Act (AIDA)
Canada is introducing AIDA, expected in 2025, to regulate high-risk AI systems.
Key Features of AIDA:
- High-Risk AI Governance: AI systems affecting health, employment, and finance must meet fairness standards.
- Accountability Measures: AI providers must ensure human oversight.
- Privacy & Transparency Rules: AI-powered decisions must be explainable to users.
Impact on Organizations:
- Businesses using AI in regulated sectors (finance, healthcare) will face strict compliance requirements.
- AI companies must register high-risk AI systems with Canadian regulators.
D. Asia-Pacific AI Governance Initiatives
The Asia-Pacific region has varied AI governance approaches, balancing regulation and innovation.
1. China’s AI Regulations: Strict Controls on AI Use
China has some of the world’s strictest AI regulations, focusing on:
- AI Transparency Laws: Deepfake and recommendation algorithms must be clearly labeled.
- Data Privacy Regulations: AI must comply with China’s Personal Information Protection Law (PIPL).
- AI Censorship Rules: AI cannot produce content against government policies.
Impact on Organizations:
- Companies operating in China must disclose AI algorithms to regulators.
- AI content moderation is strictly enforced.
2. Japan & South Korea: Pro-Innovation AI Policies
Japan and South Korea emphasize AI ethics guidelines rather than strict regulations.
- Japan’s AI Ethics Guidelines: Encourage AI fairness and transparency without legal penalties.
- South Korea’s AI Regulatory Sandbox: Allows companies to test AI innovations under relaxed rules.
Impact on Organizations:
- Companies face fewer restrictions but must follow AI fairness and transparency best practices.
2. Compliance Strategies for Organizations
To navigate AI governance regulations, organizations should adopt proactive compliance strategies.
A. Conduct AI Risk Assessments
- Identify AI use cases that fall under high-risk regulatory categories.
- Evaluate AI model fairness, security, and explainability.
- Implement impact assessments (e.g., GDPR Data Protection Impact Assessment for AI).
B. Build AI Transparency & Explainability Measures
- Ensure AI decisions are auditable and interpretable.
- Use explainable AI (XAI) tools to help users understand AI decisions.
- Provide clear documentation on AI model training and data sources.
C. Strengthen Data Governance for AI Compliance
- Ensure AI training data follows privacy laws (e.g., GDPR, CCPA, PIPL).
- Implement bias detection tools to audit training datasets.
- Maintain data lineage records to trace AI decision-making.
D. Establish AI Governance Committees
- Create AI ethics boards to oversee AI policies and regulatory compliance.
- Assign compliance officers to monitor AI governance risks.
- Regularly update internal AI policies to align with new regulations.
E. Partner with Regulatory and Industry Groups
- Engage with AI governance organizations (e.g., OECD AI Policy Observatory).
- Participate in AI regulatory discussions to stay ahead of compliance changes.
- Work with third-party auditors to ensure AI compliance certification.
Accountability Mechanisms in AI Governance
As artificial intelligence (AI) becomes an integral part of decision-making processes across industries, ensuring accountability is crucial. Without well-defined mechanisms, AI systems can operate in ways that lead to unintended consequences—bias in hiring, errors in medical diagnoses, or even financial fraud. To maintain trust, transparency, and ethical AI deployment, robust accountability mechanisms must be in place.
Defining Roles and Responsibilities
One of the fundamental steps in AI governance is establishing clear roles and responsibilities. AI is rarely developed, deployed, and maintained by a single entity or individual. Instead, it involves a complex web of stakeholders, including:
- Developers and Engineers – Responsible for coding, training, and testing AI models to ensure they function as intended. They play a key role in identifying biases and vulnerabilities.
- Data Scientists – Oversee data integrity, ensuring AI systems are trained on diverse, representative datasets to prevent discriminatory outcomes.
- Business Leaders and Executives – Make strategic decisions on AI deployment and bear ultimate responsibility for ethical considerations, risk management, and compliance.
- Regulators and Policymakers – Set the legal framework and industry guidelines that dictate how AI should be developed and used.
- End-Users – Provide feedback on AI performance and raise concerns when AI systems behave unexpectedly or unfairly.
Clearly defining these roles prevents accountability gaps where no one takes responsibility for AI failures. It also ensures that when AI systems go wrong, corrective actions can be taken swiftly.
Role of Audits and Incident Response Plans
Even with well-defined roles, AI systems can still produce unintended results. That’s where audits and incident response plans come into play.
Audits: Ensuring Transparency and Compliance
Regular AI audits—both internal and external—help assess an AI system’s fairness, reliability, and compliance with regulations. These audits focus on:
- Bias detection – Identifying and mitigating biases in AI outputs.
- Performance evaluation – Measuring AI accuracy and effectiveness over time.
- Security assessments – Checking for vulnerabilities that could be exploited by cyber threats.
- Regulatory compliance – Ensuring AI adheres to laws like GDPR, the AI Act, or industry-specific guidelines.
Companies like Google and Microsoft have dedicated AI ethics teams that conduct frequent reviews to detect and address potential risks. In some cases, independent third-party audits add an extra layer of scrutiny.
Incident Response Plans: Preparing for AI Failures
AI failures can have significant real-world consequences. A misclassified loan application, a self-driving car accident, or an AI-powered chatbot spreading misinformation—all of these scenarios require immediate action.
An effective AI incident response plan should include:
- Detection and Reporting – A mechanism for quickly identifying AI-related issues, whether through internal monitoring systems or user reports.
- Investigation and Containment – A dedicated team to assess the problem, stop further harm, and determine the root cause.
- Correction and Learning – Implementing fixes, updating AI models, and ensuring lessons learned are integrated into future AI development.
- Communication and Transparency – Informing stakeholders, including regulators and the public, about the incident and corrective measures taken.
By having structured audits and incident response plans, organizations can mitigate risks, foster trust, and ensure AI systems operate responsibly.
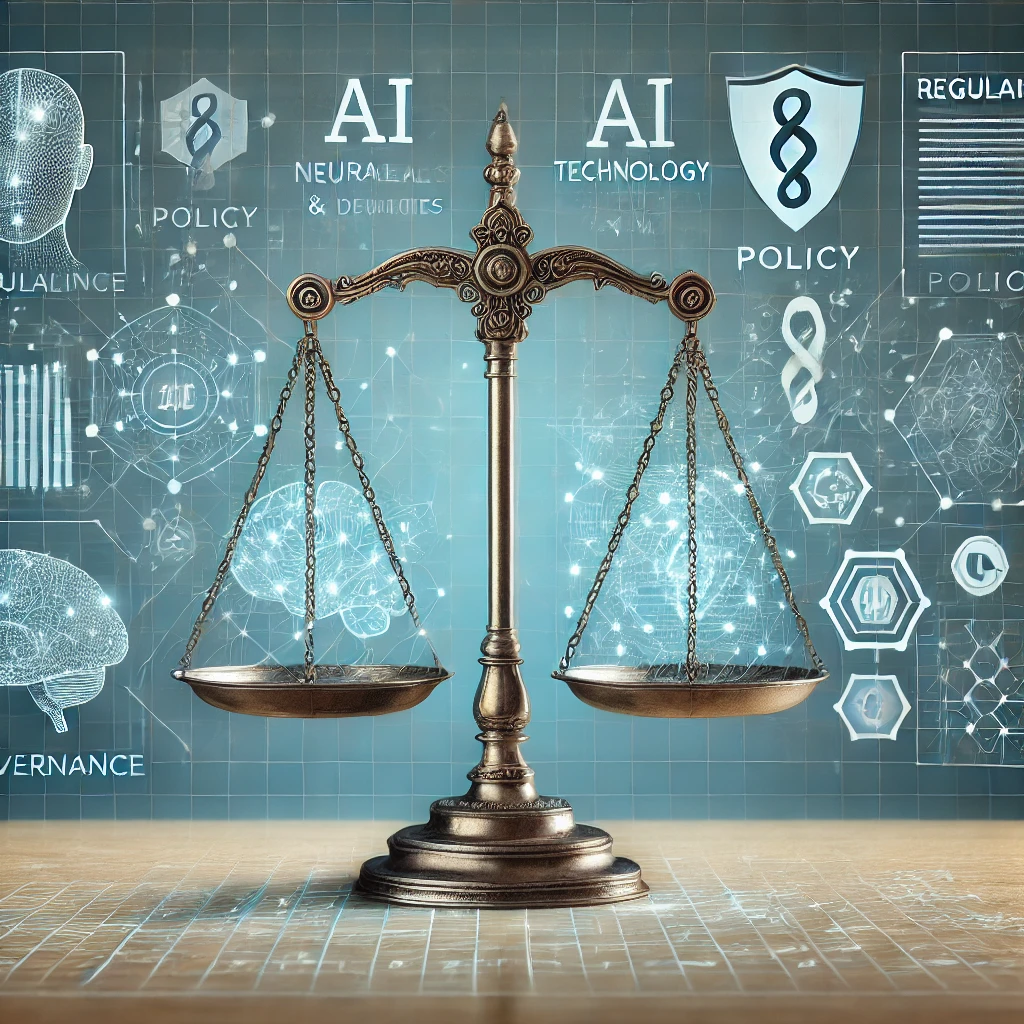
Ensuring Transparency and Explainability in AI Systems
As artificial intelligence (AI) becomes more embedded in everyday life—powering everything from healthcare diagnostics to financial decisions—one critical challenge remains: transparency. Users, businesses, and regulators need to understand how AI systems make decisions to trust them. However, AI models, particularly complex machine learning algorithms, often function as “black boxes,” making it difficult to explain their reasoning.
Transparency and explainability are not just ethical imperatives; they are essential for regulatory compliance, user trust, and business success. So how can organizations ensure AI systems remain transparent and explainable? Let’s explore key strategies.
Designing Transparent Systems
Building transparency into AI systems starts at the design stage. Organizations must prioritize explainability rather than treating it as an afterthought. Here’s how:
1. Choosing the Right AI Model
Some AI models are inherently more interpretable than others. For example:
- Linear regression and decision trees provide clear, understandable explanations of how inputs lead to outputs.
- Neural networks and deep learning models, while powerful, are often opaque and harder to interpret.
When possible, businesses should favor interpretable models, especially for high-stakes applications like healthcare, hiring, and criminal justice. If complex models are necessary, additional techniques must be used to explain their outputs.
2. Incorporating Explainability Techniques
For AI models that are not inherently transparent, developers can implement explainability tools such as:
- SHAP (Shapley Additive Explanations) – Assigns a value to each feature in an AI model’s decision, showing its impact.
- LIME (Local Interpretable Model-Agnostic Explanations) – Creates simplified, interpretable models that approximate complex AI decisions.
- Counterfactual Explanations – Provides “what-if” scenarios to show how changing inputs would affect outcomes.
These techniques help users understand AI decisions, reducing distrust and skepticism.
3. Embedding Ethical AI Principles
Developers should follow ethical AI guidelines, such as:
- Data transparency – Clearly documenting what data the AI model is trained on to identify biases.
- Fairness checks – Running AI models through fairness tests to detect discrimination.
- User-centric design – Creating AI that prioritizes human oversight and allows for manual intervention when needed.
By integrating these principles, organizations can prevent unintended biases and ensure AI serves users fairly.
Communication Strategies for Stakeholders
Even the most transparent AI system is useless if its insights are not effectively communicated. AI decisions impact multiple stakeholders—customers, regulators, employees, and executives—each with different levels of technical understanding.
1. Simplifying AI Explanations for End-Users
Users need to understand how AI systems make decisions, especially when those decisions affect their lives (e.g., loan approvals or job screening). Organizations should:
- Use plain language to explain why a decision was made.
- Offer visual explanations, such as graphs or interactive models, to break down complex AI outputs.
- Provide actionable recourse, explaining what users can do if they disagree with an AI-driven decision.
2. Transparent AI Policies for Regulators
Regulatory bodies, such as the EU’s AI Act and the U.S. Algorithmic Accountability Act, are enforcing stricter AI transparency rules. Companies must:
- Maintain detailed documentation of AI decision-making processes.
- Conduct regular audits to ensure compliance.
- Disclose AI usage in critical applications, such as hiring or credit scoring, where fairness and accountability are essential.
3. Internal AI Training for Employees
Employees interacting with AI should understand how it works. Companies should:
- Train customer service teams to explain AI decisions to users.
- Educate HR and hiring teams on AI-driven recruitment biases.
- Ensure executives and decision-makers understand AI’s capabilities and limitations.
By educating internal teams, organizations can foster a culture of responsible AI use.
Tools and Technologies Supporting Transparency
A variety of tools and technologies can help organizations enhance AI transparency.
1. Open-Source AI Transparency Tools
Several open-source frameworks help make AI more explainable:
- IBM AI Fairness 360 – Analyzes AI models for bias and fairness.
- Google’s What-If Tool – Allows users to explore AI model behavior by tweaking inputs.
- Microsoft’s InterpretML – Provides interpretability techniques for machine learning models.
These tools give developers greater insight into how AI systems function and where potential issues may arise.
2. AI Monitoring and Governance Platforms
AI governance tools can track AI decisions in real time, flagging anomalies and biases. Platforms such as Fiddler AI, TruEra, and Arthur AI help organizations:
- Monitor AI models for drift and bias.
- Provide explainability reports for regulators.
- Detect unintended AI behaviors before they cause harm.
3. Blockchain for AI Transparency
Blockchain technology is being explored as a way to improve AI accountability. By recording AI decisions on an immutable ledger, companies can:
- Ensure AI decision logs are tamper-proof.
- Create audit trails for regulators.
- Increase trust in automated processes.
While still an emerging field, blockchain could play a significant role in AI transparency in the future.
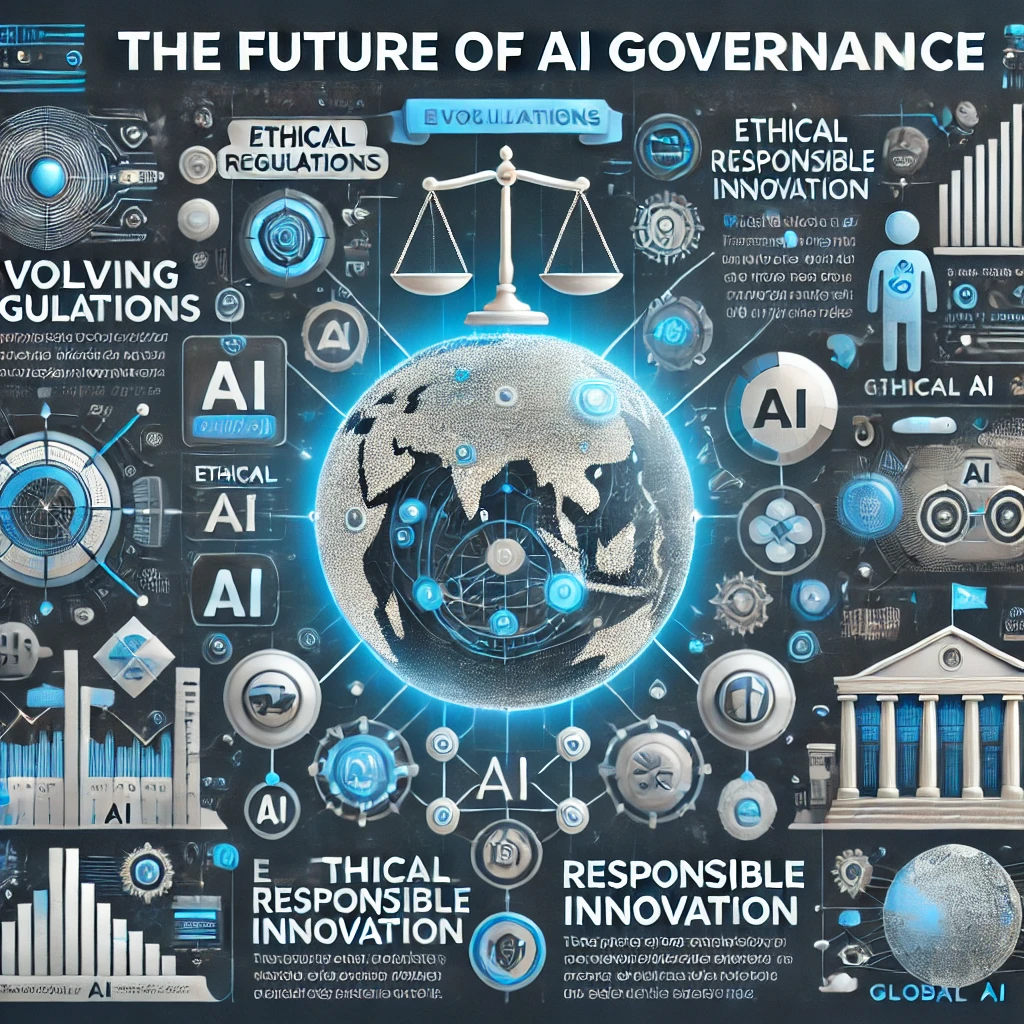
The Future of AI Governance
Artificial intelligence (AI) is evolving at an unprecedented pace, reshaping industries, economies, and societies. However, as AI becomes more powerful, so do the risks associated with its misuse, bias, and unintended consequences. To ensure AI is developed and deployed responsibly, governance structures must keep up with technological advancements.
Emerging Trends and Challenges
AI governance is at a crossroads, with several key trends shaping its future:
1. Stricter Regulations and Compliance Requirements
Governments worldwide are tightening AI regulations to address issues like bias, misinformation, and privacy violations. Examples include:
- The EU AI Act – A landmark legislation that categorizes AI systems by risk and imposes strict compliance measures for high-risk applications like facial recognition and healthcare.
- The U.S. AI Bill of Rights – A policy framework outlining principles for ethical AI use, emphasizing transparency, fairness, and user privacy.
- China’s AI Regulations – A government-led approach enforcing strict oversight, particularly on AI-generated content and deepfakes.
Companies will need to adapt to these evolving laws, ensuring their AI models comply with region-specific regulations.
2. AI Ethics and Responsible AI Initiatives
Beyond legal compliance, organizations are prioritizing ethical AI to maintain public trust. Leading tech companies are investing in:
- Bias detection and mitigation to prevent discriminatory outcomes.
- Explainable AI (XAI) to enhance transparency in AI decision-making.
- Human-AI collaboration to ensure AI augments human capabilities rather than replacing critical decision-making roles.
These initiatives are becoming industry standards, influencing future AI governance frameworks.
3. Challenges in AI Accountability
One of the biggest obstacles in AI governance is assigning responsibility when things go wrong. Key challenges include:
- Opaque AI models – Many AI systems, particularly deep learning models, function as “black boxes,” making it difficult to understand their decision-making process.
- Liability issues – If an AI-powered system makes a harmful decision (e.g., an autonomous vehicle accident), determining accountability—whether it falls on the developer, the organization, or the user—remains a legal gray area.
- Global inconsistency in AI laws – Different countries have different AI regulations, making compliance challenging for multinational companies.
Addressing these challenges requires a global, coordinated effort.
Global Efforts for Unified AI Governance
While nations are developing their own AI regulations, there is growing recognition that AI governance must be global. AI systems don’t recognize borders, and unregulated AI in one country can have consequences worldwide.
Here’s how governments and organizations are working toward unified AI policies:
1. Multilateral AI Governance Initiatives
Several global organizations are spearheading AI governance discussions:
- The United Nations (UN) Advisory Body on AI – Established to develop global AI governance recommendations, ensuring AI benefits all of humanity.
- The OECD AI Principles – A set of international guidelines promoting trustworthy AI, adopted by 46 countries.
- The G7 AI Code of Conduct – A voluntary commitment by leading economies to ensure AI safety, transparency, and accountability.
These initiatives aim to align AI policies across different nations, fostering collaboration rather than fragmentation.
2. Public-Private Partnerships in AI Governance
Governments and corporations are working together to shape responsible AI use. Examples include:
- The AI Partnership on AI (PAI) – A coalition of companies (Google, Microsoft, IBM) and NGOs working on ethical AI standards.
- The World Economic Forum’s AI Governance Alliance – Bringing together policymakers and business leaders to develop ethical AI frameworks.
Such partnerships ensure AI governance isn’t dictated solely by governments or corporations but is a collective effort.
3. Standardization Efforts by Tech Leaders
AI companies are recognizing the importance of self-regulation and are taking proactive steps:
- OpenAI and Microsoft are advocating for AI safety research and responsible deployment of large-scale AI models.
- Google’s AI Principles guide its AI development, focusing on fairness, accountability, and transparency.
- Meta’s AI Transparency Reports provide insights into how AI is used in content moderation and social media algorithms.
These voluntary commitments set industry benchmarks for responsible AI governance.
The Potential for an International AI Governance Framework
Given AI’s global impact, many experts argue that a binding international AI governance framework—similar to climate agreements or nuclear treaties—may be necessary. But is this achievable?
1. The Case for a Global AI Treaty
A legally binding international AI framework could:
- Set universal safety and ethical standards for AI development and deployment.
- Create mechanisms for cross-border AI risk management, preventing misuse of AI in warfare, cyberattacks, or deepfake propaganda.
- Ensure equitable AI access, preventing technological monopolies by a few powerful nations or corporations.
2. Obstacles to Global AI Governance
Despite the benefits, establishing a global AI governance framework faces significant hurdles:
- Geopolitical competition – The U.S., China, and the EU have different AI priorities, making consensus difficult.
- Technological sovereignty concerns – Countries may resist global AI rules that limit their ability to innovate or control their AI industries.
- Enforcement challenges – Without a global AI regulatory body, ensuring compliance across different legal systems would be complex.
3. A Realistic Path Forward: Regional AI Governance Blocks
Instead of a single global treaty, we may first see regional AI governance frameworks emerge. For example:
- The EU AI Act could become a global standard, much like GDPR influenced global data privacy laws.
- The G7 AI Code of Conduct could expand to include more countries, setting the foundation for a broader agreement.
- The BRICS nations (Brazil, Russia, India, China, South Africa) might develop their own AI regulatory framework, influencing emerging markets.
These regional frameworks could eventually converge into a more unified global AI governance model.
Monitoring and Continuous Improvement in AI Governance
AI governance is not a one-time policy implementation—it’s a continuous process that requires regular monitoring, evaluation, and adaptation. As AI systems evolve, so do the risks, ethical considerations, and regulatory landscapes surrounding them. Without effective monitoring and mechanisms for continuous improvement, even the best-intended AI governance frameworks can become outdated, ineffective, or even counterproductive.
Key Performance Metrics for AI Governance
To effectively govern AI systems, organizations must track measurable indicators that assess the performance, fairness, and compliance of their AI models. These metrics help organizations identify risks early, maintain accountability, and ensure AI systems operate as intended.
1. Accuracy and Reliability
One of the fundamental measures of AI performance is its accuracy. AI models should be evaluated regularly to ensure they produce consistent and reliable outputs. Key metrics include:
- Precision and recall – Used in classification models to measure how accurately AI identifies relevant results.
- False positive/false negative rates – Important in high-risk applications like fraud detection or medical diagnostics, where incorrect classifications can have severe consequences.
- Model drift detection – AI models may degrade over time as real-world conditions change, so continuous testing is necessary.
2. Fairness and Bias Mitigation
AI should not discriminate against individuals based on race, gender, age, or other protected attributes. Measuring fairness involves:
- Disparate impact analysis – Checking if AI decisions disproportionately affect certain groups.
- Equalized odds – Ensuring AI has similar false positive/negative rates across different demographic groups.
- Fairness-aware metrics (e.g., Demographic Parity) – Ensuring AI models distribute outcomes equitably.
3. Transparency and Explainability
AI systems must be interpretable, particularly in high-stakes applications. Organizations should track:
- Explainability scores – Measuring how well AI decisions can be understood using tools like SHAP (Shapley Additive Explanations) and LIME (Local Interpretable Model-Agnostic Explanations).
- User comprehension rates – Assessing whether end-users understand AI-driven decisions.
- Documentation completeness – Ensuring AI models have clear documentation on training data, assumptions, and limitations.
4. Security and Robustness
AI systems must be resilient to cyber threats, adversarial attacks, and unintended manipulations. Important security metrics include:
- Adversarial robustness – Testing AI models against adversarial inputs to see if they can be tricked.
- Data integrity checks – Ensuring training data hasn’t been tampered with or poisoned.
- Incident response time – Measuring how quickly AI governance teams can respond to and resolve security breaches.
5. Regulatory Compliance
With AI regulations increasing globally, organizations must track:
- Compliance adherence rates – Measuring whether AI models meet legal and industry-specific regulations (e.g., GDPR, the EU AI Act, or the Algorithmic Accountability Act).
- Audit frequency and findings – Ensuring AI systems undergo regular internal and external audits.
- User complaint resolution time – How quickly AI-related concerns raised by users are addressed.
By continuously tracking these metrics, organizations can proactively detect risks and make necessary governance adjustments.
Feedback Loops and Iterative Improvements
Effective AI governance is not static—it relies on continuous learning and adaptation. Feedback loops help organizations refine AI systems, improve fairness, and enhance transparency.
1. Internal Feedback Mechanisms
Organizations should establish structured processes to evaluate AI performance and governance effectiveness:
- AI Governance Committees – Cross-functional teams that regularly review AI performance metrics and make policy recommendations.
- Developer and Data Scientist Reviews – Encouraging AI engineers to identify biases, errors, or unexpected behaviors in models.
- Periodic Model Retraining – Updating AI models based on new data and evolving societal norms.
2. External Feedback from Users and Stakeholders
AI systems impact users, customers, and society at large, making external feedback essential:
- User Feedback Surveys – Collecting input from users on AI-driven decisions and their experiences with AI-powered services.
- Regulator and Watchdog Reports – Addressing concerns raised by regulatory agencies or AI ethics organizations.
- Independent Audits and Red-Teaming – External experts testing AI systems for vulnerabilities and biases.
Companies like Google, OpenAI, and Microsoft have implemented AI “red teams”—dedicated groups that stress-test AI models to identify weaknesses and ethical risks.
3. Incident Response and Post-Mortem Analysis
When AI systems fail—whether through bias, security breaches, or unexpected behaviors—it’s critical to conduct post-mortem analysis and apply lessons learned:
- Root cause analysis – Identifying why an AI model failed or produced unintended consequences.
- Corrective action plans – Implementing changes to prevent similar failures in the future.
- Transparency in communication – Informing stakeholders about incidents and governance updates.
By integrating these feedback loops, AI governance remains a living process that evolves with changing risks and societal expectations.
Adapting Governance to Technological Advancements
AI technology is evolving rapidly, introducing new challenges that governance frameworks must address. Here’s how organizations can stay ahead:
1. Keeping Up with Emerging AI Trends
AI governance must anticipate advancements such as:
- Generative AI – As AI-generated content (e.g., deepfakes, synthetic text) becomes more sophisticated, governance frameworks must include guidelines for responsible use.
- Autonomous AI Agents – AI systems capable of making complex decisions with minimal human oversight require new accountability structures.
- Quantum AI – The rise of quantum computing could disrupt encryption, privacy, and AI security, necessitating new governance strategies.
2. Updating Governance Policies with AI Evolution
Governance frameworks must evolve alongside technological advancements. Best practices include:
- Regular AI policy updates – Companies should review governance policies at least annually to ensure alignment with emerging risks.
- Collaboration with AI Research Communities – Engaging with universities, think tanks, and AI ethics organizations to stay informed.
- Scenario Planning for Future Risks – Organizations should conduct AI impact assessments to predict potential risks before they arise.
3. Investing in AI Governance Infrastructure
To support continuous improvement, companies should invest in:
- AI Monitoring Platforms – Tools like Fiddler AI, Arthur AI, and IBM’s AI Fairness 360 can provide real-time governance insights.
- Automated Compliance Systems – AI-driven compliance tools can streamline regulatory adherence.
- AI Ethics and Governance Training – Employees across departments should receive training on AI risks and governance best practices.
By staying proactive, organizations can ensure their AI governance remains relevant, ethical, and aligned with both technological advancements and societal expectations.
Securing AI Systems: Protecting AI from Risks and Threats
As AI systems become more advanced and integrated into critical industries—healthcare, finance, cybersecurity, and national security—the risks associated with AI misuse, manipulation, and failure grow exponentially. AI security is no longer just about protecting data; it involves ensuring model integrity, mitigating biases, preventing adversarial attacks, and safeguarding AI against malicious threats.
Without robust security measures, AI can become a vulnerability rather than an asset. This post explores how organizations can build risk frameworks, implement mitigation strategies, and defend AI systems from cyber threats.
Building Risk Frameworks and Mitigation Strategies
A structured risk management framework is essential for identifying vulnerabilities in AI systems and developing proactive defenses. A strong AI security strategy includes:
1. Identifying AI-Specific Risks
AI security risks differ from traditional IT risks. Key concerns include:
- Adversarial Attacks – Malicious actors manipulate AI inputs to deceive models (e.g., tricking facial recognition systems or causing misclassifications in autonomous vehicles).
- Data Poisoning – Attackers inject biased or harmful data into AI training datasets, corrupting model outputs.
- Model Inversion Attacks – Hackers extract sensitive training data from AI models, compromising privacy.
- Model Theft – Competitors or malicious entities steal proprietary AI models through API scraping or insider threats.
- Bias and Ethical Failures – Poorly trained AI models can amplify discrimination, leading to reputational damage and regulatory penalties.
2. Implementing AI Risk Assessment Frameworks
Organizations must systematically assess AI risks before deployment. Some industry-standard frameworks include:
- NIST AI Risk Management Framework (AI RMF) – A U.S. government framework focusing on AI trustworthiness, fairness, and resilience.
- ISO/IEC 23894 AI Security Standards – International AI security guidelines to protect against vulnerabilities.
- The AI Trustworthiness Framework (by the OECD) – A global guideline for ensuring AI safety, security, and accountability.
By using these frameworks, organizations can categorize AI risks and develop tailored security measures.
3. Mitigation Strategies for AI Security Risks
To protect AI systems, organizations should adopt the following best practices:
a) Data Security and Robustness
- Data Validation Pipelines – Implement automated tools to detect anomalies or poisoned data in training datasets.
- Encryption and Differential Privacy – Use techniques like homomorphic encryption and federated learning to prevent unauthorized data access.
- Synthetic Data for Training – Reduces reliance on real-world sensitive data, lowering the risk of privacy breaches.
b) Model Security and Adversarial Defense
- Adversarial Training – Expose AI models to adversarial examples during training to improve resilience.
- Anomaly Detection Systems – Continuously monitor AI decisions for suspicious patterns that could indicate tampering.
- Model Watermarking – Embedding unique identifiers in AI models to detect theft or unauthorized modifications.
c) AI Governance and Human Oversight
- Explainability and Transparency Tools – Use SHAP, LIME, and counterfactual analysis to understand AI decision-making.
- Regular AI Security Audits – Conduct penetration testing and red-teaming exercises to uncover weaknesses.
- Human-in-the-Loop (HITL) Systems – Require human review for high-risk AI decisions, reducing the impact of model failures.
By integrating these mitigation strategies, organizations can create resilient AI systems that are secure, reliable, and accountable.
Safeguarding AI Against Malicious Threats
AI is increasingly targeted by cybercriminals, nation-state actors, and unethical businesses. Here’s how organizations can defend their AI systems against malicious threats:
1. Defending Against Adversarial AI Attacks
Adversarial attacks are designed to manipulate AI behavior. Common types include:
- Evasion Attacks – Attackers slightly modify input data to trick AI models into making incorrect predictions (e.g., altering stop signs to fool self-driving cars).
- Backdoor Attacks – Attackers inject hidden patterns into training data so AI behaves normally during testing but maliciously under specific conditions.
Defensive Measures:
- Robust AI Model Training – Use adversarial training techniques to expose AI models to attack scenarios.
- AI Firewalls – Deploy AI-specific security tools that detect and block adversarial inputs in real time.
- Explainable AI (XAI) Audits – Continuously analyze AI decision-making to detect anomalies caused by attacks.
2. Preventing AI Data Poisoning and Model Manipulation
Attackers can compromise AI models by tampering with their training data, leading to biased or unsafe outputs.
Defensive Measures:
- Data Provenance Verification – Track the source of all training data to ensure its authenticity.
- Secure AI Model Updates – Use cryptographic signatures to validate updates before deployment.
- Decentralized Training Techniques – Use federated learning, which prevents central data storage and reduces attack vectors.
3. Protecting AI APIs and Cloud-Based AI Services
Many organizations deploy AI models through APIs and cloud platforms, making them vulnerable to cyberattacks.
Defensive Measures:
- Rate Limiting & Access Control – Prevent unauthorized access by restricting API usage based on authentication levels.
- AI Model Throttling – Limit excessive AI model queries to prevent model extraction attacks.
- Secure Cloud AI Environments – Use zero-trust security models for cloud-based AI deployments.
4. Addressing AI Supply Chain Risks
AI systems rely on open-source libraries, third-party datasets, and pre-trained models, all of which introduce potential security vulnerabilities.
Defensive Measures:
- Supply Chain Audits – Verify the security of external AI components before integration.
- Dependency Monitoring Tools – Track and update AI dependencies to patch security vulnerabilities.
- Restricted Open-Source Usage – Avoid using unverified AI models from unknown sources, reducing exposure to hidden malware or biases.
5. Preparing for AI-Powered Cyber Threats
Just as AI can be used defensively, cybercriminals are using AI for:
- Automated Phishing Attacks – AI generates highly convincing phishing emails.
- Deepfake Scams – AI-generated audio and video are used for fraud and misinformation campaigns.
- AI-Driven Malware – Cybercriminals use AI to develop self-learning malware that evades traditional security measures.
Defensive Measures:
- AI-Enhanced Threat Detection – Organizations must fight AI with AI by using machine learning-driven cybersecurity solutions.
- Real-Time Deepfake Detection – Use AI models to detect manipulated media in security-sensitive applications.
- AI-Powered Incident Response Teams – Automate security responses using AI to counter AI-driven attacks.
Conclusion
The Critical Role of AI Governance and the Path Forward
AI is transforming industries, revolutionizing decision-making, and reshaping the global economy. However, with its immense potential comes significant responsibility. AI governance is no longer optional—it is a fundamental requirement to ensure AI systems remain ethical, secure, transparent, and aligned with societal values.
Throughout this discussion, we have explored the key pillars of AI governance:
- Accountability mechanisms to define roles, responsibilities, and incident response strategies.
- Transparency and explainability to build trust with stakeholders and ensure AI decisions are interpretable.
- Security frameworks to safeguard AI from malicious threats, adversarial attacks, and data breaches.
- Monitoring and continuous improvement to track AI performance, integrate feedback loops, and adapt to evolving risks.
- Global governance efforts to establish unified regulations and frameworks for responsible AI development.
A Call to Action for Organizations and Policymakers
To ensure AI serves humanity’s best interests, organizations and policymakers must take proactive steps:
For Organizations:
- Implement AI governance frameworks that prioritize fairness, accountability, and transparency.
- Invest in security and risk management to protect AI systems from cyber threats and adversarial manipulation.
- Continuously monitor AI performance using key metrics and feedback loops to detect issues early.
- Foster a culture of ethical AI development by training employees and encouraging responsible AI innovation.
For Policymakers:
- Establish clear regulations and guidelines that promote AI safety without stifling innovation.
- Encourage global cooperation to create unified AI governance standards across nations.
- Support AI research and development that focuses on ethical, fair, and unbiased AI models.
- Hold organizations accountable for AI misuse and ensure transparent auditing processes.
The Future of AI Governance
As AI technology advances, governance must evolve alongside it. Emerging challenges—such as generative AI, autonomous systems, and AI-powered cyber threats—require dynamic and adaptive governance strategies. Collaboration between governments, businesses, academia, and civil society is essential to build AI systems that are not only powerful but also trustworthy and beneficial to humanity.
FAQs
The time to act is now. By prioritizing responsible AI governance today, we can harness AI’s potential while safeguarding society from its risks. The future of AI depends not just on innovation, but on how well we govern it.
1. What is AI governance?
AI governance refers to the policies, frameworks, and regulations that guide the responsible development, deployment, and use of artificial intelligence. It ensures that AI systems operate ethically, transparently, and safely while minimizing risks related to bias, privacy, and security.
2. Why is AI governance important?
AI governance is crucial because AI systems influence critical decisions in healthcare, finance, law enforcement, and other sectors. Without proper governance, AI can lead to unintended consequences such as biased decision-making, security vulnerabilities, and privacy violations. Governance frameworks help ensure AI is used responsibly and in alignment with societal values.
3. What are the key principles of AI governance?
The core principles of AI governance include:
- Transparency – AI decisions should be understandable and explainable.
- Accountability – Organizations must take responsibility for AI-driven outcomes.
- Fairness & Bias Mitigation – AI should not discriminate against individuals or groups.
- Privacy & Security – AI must protect user data and be resilient against cyber threats.
- Continuous Monitoring – AI systems should be regularly evaluated and improved.
4. How does AI governance differ from AI ethics?
AI ethics focuses on the moral principles and values that should guide AI development, such as fairness and human rights. AI governance, on the other hand, involves the practical implementation of these ethical principles through laws, regulations, and organizational policies. In short, AI ethics defines the “why,” while AI governance focuses on the “how.”
5. What are the biggest challenges in AI governance?
Some of the major challenges include:
- Lack of standardized regulations – AI laws vary across countries, making global governance difficult.
- Bias in AI models – Ensuring fairness in AI requires constant oversight.
- Security risks – AI is vulnerable to cyberattacks and adversarial manipulation.
- Balancing innovation and regulation – Too much regulation can stifle AI advancements, while too little can lead to ethical risks.
6. Who is responsible for AI governance?
AI governance is a shared responsibility among multiple stakeholders:
- Governments & policymakers – Set regulations and enforce compliance.
- Businesses & developers – Implement governance frameworks in AI products.
- Academia & researchers – Study AI risks and propose best practices.
- Civil society & advocacy groups – Ensure AI systems respect human rights and public interests.
7. What role do audits and accountability play in AI governance?
Audits help ensure AI systems comply with ethical and legal standards by evaluating their fairness, transparency, and performance. Organizations should also establish clear accountability structures so that AI-related mistakes or harms can be addressed and rectified.
8. How can organizations implement AI governance?
Organizations can implement AI governance by:
- Developing internal policies that align with ethical AI principles.
- Conducting regular AI audits to assess risks and biases.
- Using explainable AI (XAI) tools to enhance transparency.
- Establishing an AI ethics committee to oversee responsible AI use.
9. Are there global efforts to regulate AI?
Yes, multiple global initiatives aim to regulate AI responsibly:
- The EU AI Act – A comprehensive regulatory framework for AI governance in the European Union.
- The OECD AI Principles – International guidelines promoting trustworthy AI.
- The U.S. AI Bill of Rights – A set of AI governance principles proposed by the U.S. government.
- The United Nations AI Advisory Board – Focused on global cooperation for responsible AI.
10. How will AI governance evolve in the future?
AI governance will likely become more standardized across countries, incorporating more stringent regulations, advanced security measures, and greater transparency requirements. As AI technology advances, governance frameworks will need to adapt to emerging risks, such as deepfakes, autonomous AI systems, and quantum AI threats.
11. How does AI governance address bias and fairness in AI systems?
AI governance frameworks include guidelines and best practices to identify, reduce, and prevent bias in AI systems. This involves:
- Using diverse and representative training datasets to avoid systemic discrimination.
- Conducting bias audits and fairness testing to detect and correct unfair patterns.
- Implementing explainable AI (XAI) techniques to understand how decisions are made.
- Requiring human oversight in high-risk AI applications to ensure fair outcomes.
12. What is the role of transparency and explainability in AI governance?
Transparency and explainability ensure that AI decisions are understandable to users, regulators, and stakeholders. AI governance promotes transparency by:
- Providing clear documentation on how AI models function.
- Developing interpretable AI models that allow users to see how inputs affect outputs.
- Using tools like SHAP and LIME to explain complex AI decisions.
- Mandating disclosure policies that inform users when AI is making decisions about them.
13. How do governments regulate AI governance?
Governments regulate AI governance through a combination of:
- Legislation – Laws such as the EU AI Act and China’s AI regulations set clear legal requirements for AI safety and fairness.
- Guidelines and frameworks – Organizations like OECD, NIST, and the UN create international AI governance principles.
- Compliance and enforcement – Government agencies monitor AI systems and impose penalties for violations.
14. What industries are most affected by AI governance?
AI governance is critical in industries where AI has a direct impact on people’s lives, including:
- Healthcare – AI is used for diagnostics, treatment recommendations, and medical research, requiring strict ethical oversight.
- Finance – AI-driven credit scoring, fraud detection, and algorithmic trading must be fair and transparent.
- Law enforcement – AI surveillance and facial recognition technologies raise privacy and human rights concerns.
- Employment and HR – AI-powered hiring tools must prevent discrimination in recruitment and promotions.
15. What happens when AI governance is weak or absent?
Without strong AI governance, organizations risk:
- Biased decision-making that leads to discrimination and unfair treatment.
- Security vulnerabilities that expose AI systems to cyberattacks and manipulation.
- Loss of public trust in AI-powered systems, making adoption difficult.
- Legal and financial penalties from regulatory violations and lawsuits.
16. How does AI governance impact AI safety and security?
AI governance ensures that AI systems are resilient against threats such as adversarial attacks, data poisoning, and deepfake manipulations. It promotes:
- Robust security frameworks to protect AI models and data.
- Incident response plans to quickly address AI failures or breaches.
- Continuous monitoring to detect security threats in real time.
17. Can AI governance prevent the misuse of AI in warfare and surveillance?
AI governance can establish international agreements to regulate AI use in military applications, similar to arms control treaties. However, enforcing such regulations requires global cooperation among governments, tech companies, and policymakers. Some organizations, like the United Nations and the Partnership on AI, advocate for ethical AI use in warfare and surveillance.
18. How does AI governance support innovation rather than hinder it?
Well-designed AI governance frameworks balance innovation with responsibility by:
- Providing clear regulations that prevent AI misuse without stifling development.
- Encouraging ethical AI research to build trustworthy and fair AI models.
- Creating regulatory sandboxes where companies can test AI systems in a controlled environment before full deployment.
19. How can businesses comply with AI governance regulations?
Businesses can comply with AI governance by:
- Conducting regular AI risk assessments to identify potential ethical and legal risks.
- Following industry standards and best practices such as ISO/IEC AI security standards.
- Training employees on AI ethics and compliance.
- Establishing an AI ethics board to oversee AI development and deployment.
20. What is the future of AI governance?
The future of AI governance will likely involve:
- More global cooperation to create standardized AI regulations across countries.
- Stronger enforcement mechanisms to hold companies accountable for AI misuse.
- Advancements in AI auditing tools to improve AI transparency and fairness.
- AI self-regulation mechanisms where AI systems can auto-detect and correct biases.